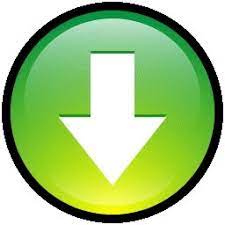
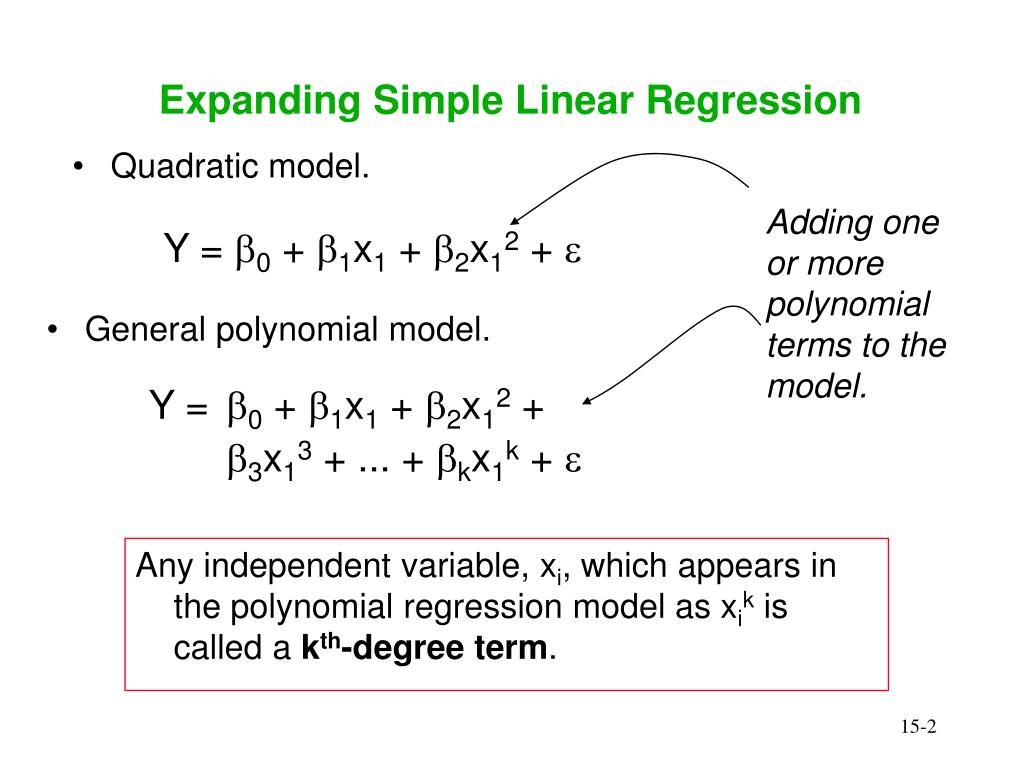


There are five assumptions associated with the linear regression model (these are called the Gauss-Markov assumptions): To be able to get reliable estimators for the coefficients and to be able to interpret the results from a random sample of data, we need to make model assumptions. In simple linear regression, we essentially predict the value of the dependent variable yi using the score of the independent variable xi, for observation i. β0 is the intercept (a constant term) and β1 is the gradient. Here, β0 and β1 are the coefficients (or parameters) that need to be estimated from the data. Where the subscript i refers to a particular observation (there are n data points in total). In this way, the linear regression model takes the following form: To capture all the other factors, not included as independent variable, that affect the dependent variable, the disturbance term is added to the linear regression model. The disturbance is primarily important because we are not able to capture every possible influential factor on the dependent variable of the model. The relationship is modeled through a random disturbance term (or, error variable) ε. The linearity of the relationship between the dependent and independent variables is an assumption of the model. Linear regression is used to study the linear relationship between a dependent variable (y) and one or more independent variables ( X). As the name suggests, this type of regression is a linear approach to modeling the relationship between the variables of interest. In this article, I am going to introduce the most common form of regression analysis, which is the linear regression. By applying regression analysis, we are able to examine the relationship between a dependent variable and one or more independent variables. Regression analysis is an important statistical method for the analysis of data. That this formula minimizes the squared distancesįrom each of these points to that line.Introduction to the core concepts of simple linear regression and OLS estimation Background And it actually won't goĭirectly through that line. Going to be that exact, is going to look like that Graph it, and obviously I'm hand drawing it, so it's not To be equal to the mean of the y's, the mean of the And this is the same thingĪs negative 2/3 times negative 9/ 14. What's 49 minus 63? That's negative 14/9. Something over 9, that's the same thing as 63/9. I'm tempted to get out myĬalculator, but i'll resist the temptation. And we just have to do a littleīit of mathematics. So our slope, our optimal slopeįor our regression line, the mean of the x's is The x squareds? The first x squared is just To calculate is the mean of the x squareds.
ECONOMICS SIMPLE LINEAR REGRESSION EQUATION PLUS
To be equal to? We have 2 plus 2, which is 4. And what's this going to be? 1 plus 2 is 3, plus 4 So what's the mean of our x's? The mean of our x's is going To calculate these things ahead of time, and then Like using our formulas, which we have proven. It the best fitting regression line, which we suspect
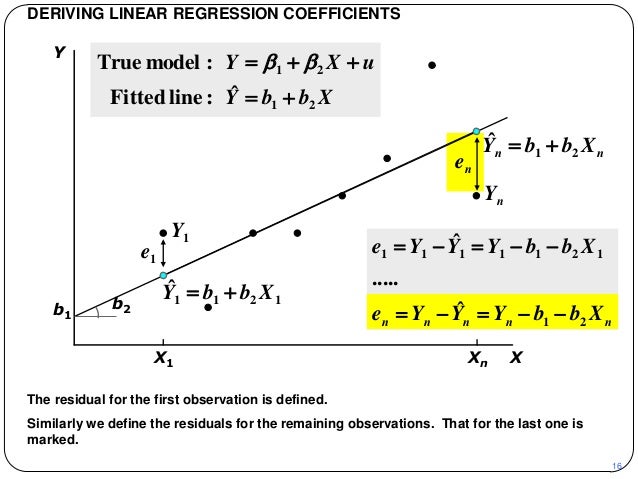
Have the point, let's do something a little bitĬrazy, 4 comma 3. Points, and I'm going to make sure that these pointsĪren't colinear. And of course, whatever you getįor m, you can then just substitute back in this Numerator and denominator by negative 1, which is same thingĪs multiplying the whole thing by 1. X squareds minus the mean of the x's squared. Written as the mean of the xy's minus the mean of x times Numerator and denominator by negative 1, you could see this Textbook, you might see this swapped around. And if this looks a littleĭifferent than what you see in your statistics class or your Really confusing, we're going to do an example of thisĪctually in a few seconds. Going to be the mean of x's times the mean of the y's minus Just rewrite it here just so we have something When you measure the error by the squared distance Slope and y-intercept of the best fitting regression line
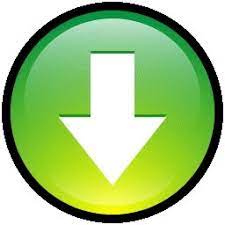